News
Key Gender Parity Metric Falls for First Time in 2 Decades
2024-03-19.jpg)
Elusive Parity: Key Gender Parity Metric Falls for First Time in 2 Decades
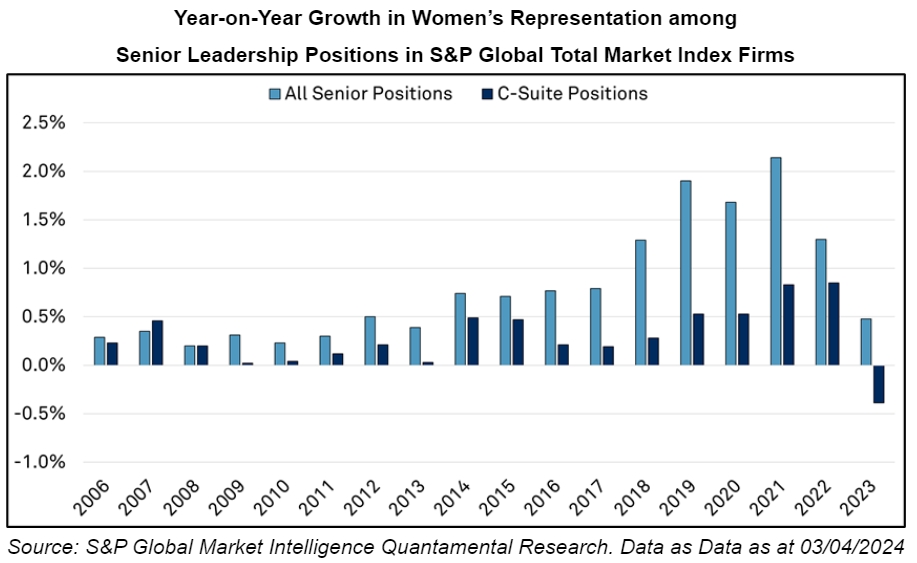
The growth in women’s representation among senior corporate positions, once a bright spot for gender parity, potentially faces an alarming turning point. Exponential growth over a decade is showing signs of losing momentum. Growth no longer appears exponential. A waning focus on diversity initiatives suggests a potential inflection point and calls our previous gender parity estimates into question.
Read the report >
Key takeaways:
● The growth in women’s representation among all senior leadership positions in the U.S. dropped to the lowest rate in more than a decade, 0.5% vs. 1.2% average.
● Across C-Suite positions, women lost seats for the first time over the study period (2005-2023). Women held just 12.2% of the ~15,000 C-suite positions across publicly traded U.S. firms in 2022. However, women retraced progress to 11.8% in 2023.
● Models of parity forecasts for senior leadership positions among U.S. firms now suggest parity 1 to 7 years later (2033 – 2042), compared to 2022 estimates.
● Natural Language Processing (NLP) of earnings call transcripts shows that publicly traded firms are spending less time on diversity and inclusion. Mentions of the topic have fallen to multi-year lows.
Read the report >
Discover the datasets used in this research:
S&P Global Professionals Dataset
The dataset includes board and company affiliations, executive biographies, standardized job functions, titles, education, and compensation for more than 4.5 million professionals going back to 1992. Data are primarily sourced from Proxy Filings DEF-14A and amendments to 10-K filings, as well as other regulatory filings and press releases.
Learn More
Machine-Readable Transcripts
This dataset aggregates data from earnings calls delivered in a machine-readable format for Natural Language Processing (NLP) applications with metadata tagging. With our NLP-ready datasets, you can skip the entire data management process and dive directly into analysis. Moreover, benefit from comprehensive metadata tagging and seamless integration with other S&P datasets.
Learn More
Related files:
NoData